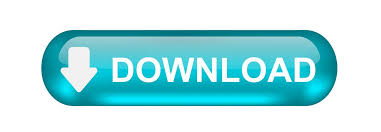
A higher number denotes higher dependency.Ĭorrelation is a statistical measure that indicates how strongly two variables are related. Basis for comparisonĬovariance is an indicator of the extent to which 2 random variables are dependent on each other. Now you will see the differences between Covariance and Correlation. When it comes to linear regression, for example, a large number of correlations indicate that the linear regression estimates will be unreliable. When checking other analyses, as a diagnostic.When excluding missing values pairwise, correlation matrices are commonly used as inputs for exploratory factor analysis, confirmatory factor analysis, structural equation models, and linear regression. As a result, a correlation matrix is used to look for a pattern in the data and determine whether the variables are highly correlated. The goal when dealing with large amounts of data is to find patterns.Applications of CorrelationĪ correlation matrix is computed for three main reasons: We will continue our learning of the covariance vs correlation differences with these applications of the correlation matrix. Rc = coefficient of concurrent deviations N = number of items ranked Coefficient of Concurrent DeviationsĬoefficient of concurrent deviations is used when you want to study the correlation in a very casual manner and there is not much need to attain precision. It measures the extent to which, as one variable increases, the other decreases. It is obtained by dividing the covariance of two variables with the product of their standard deviations.Ī rank correlation coefficient measures the degree of similarity between two variables, and can be used to assess the significance of the relation between them. This is the most common method of determining the correlation coefficient of two variables. Here are some of the most common ones: Coefficient of correlation There are a number of methods to calculate correlation coefficient. Multiple correlation: A statistical technique that uses two or more variables to predict the value of one variable.Partial Correlation: When one variable's effects are removed, the correlation between two variables is revealed in partial correlation.Simple Correlation: In simple correlation, a single number expresses the degree to which two variables are related.When variables move in the opposite direction, they are said to be negatively correlated. Positive correlation occurs when two variables move in the same direction. When an equivalent movement of another variable reciprocates the movement of one variable in some way or another during the study of two variables, the variables are said to be correlated. In statistics, correlation is a measure that determines the degree to which two or more random variables move in sequence. To perform principal component analysis, an eigen decomposition is applied to the covariance matrix To reduce the dimensions of large data sets, principal component analysis is used.The matrix is decomposed by the product of the lower matrix and its transpose. A covariance matrix helps determine the Cholesky decomposition because it is positive semi-definite. Simulating systems with multiple correlated variables is done using Cholesky decomposition.The following are the most common applications of Covariance: Let Σ(X) and Σ(Y) be the expected values of the variables, the covariance formula can be represented as: Covariance is great for defining the type of relationship, but it's terrible for interpreting the magnitude. A negative number, on the other hand, denotes negative covariance, which indicates an inverse relationship between the two variables.
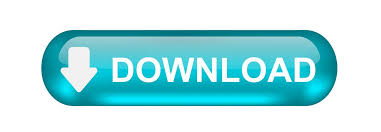